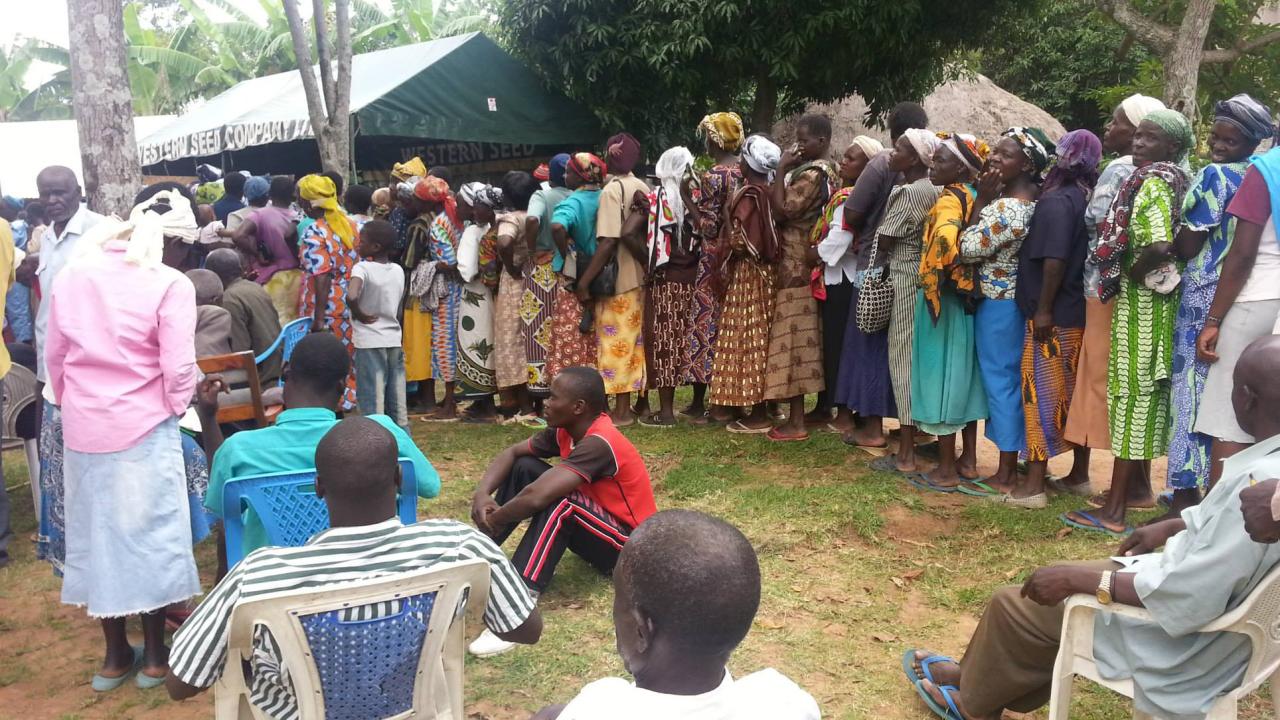
The economic and human development costs of uninsured risk have long been recognized. Recent advances in remote sensing and automated weather measurement open the door to innovative index insurance contracts that transfer correlated risk out of small farm economic systems. A small but compelling body of evidence confirms that index insurance achieves development objectives by inducing small farmers to boost their incomes by prudentially investing more in production (Ghana and Mali), and by reducing reliance on costly coping strategies in the wake of shocks (Kenya).
Despite its promise, the development of agricultural insurance markets sometimes appears stymied by high prices. While simple subsidies may be an appropriate policy response, this brief digs deeper and suggests that prices are high because of “uncertainty loadings” levied on insurance premiums because of sparse data problems that are endemic in areas that could most benefit from agricultural insurance.
By exploiting the capacity of a public reinsurance facility to operate in an uncertainty-neutral way, this brief argues that the promised impact of agricultural insurance can best and most cost-effectively be achieved by a novel public-private reinsurance partnership. Importantly, this partnership is one that could evolve over time— with the public sector eventually withdrawing— as the sparse data problem naturally resolves itself as more data are collected over time.
The Challenge of Viable Insurance Markets
While there are many challenges to the creation of viable agricultural insurance markets that promote economic development objectives, three of the most critical are:
- Contract Design: If insurance is to protect and incentivize farmers so that the kinds of gains seen in the Ghana, Mali and Kenya pilots are to be realized, contracts must have low failure rates, meaning that they almost always indemnify farmers when losses occur.
- Contract Pricing: If the insurance market is to grow, contracts need to be attractively priced given that target populations have modest income levels, are often liquidity constrained and are price sensitive. This observation does not imply that subsidies are needed, but it does mean that if prices drift much above actuarially fair prices, or if farmers see little probability of ever receiving a payout given their historical experience, then the market is unlikely to develop.
- Contract Availability: Given fixed costs of innovation, scale is critical to development of the insurance market. Uncertainty averse pricing strategies that bypass or redline areas where local data is missing will retard overall market development.
Recent pilot projects have shown that the contract design problem can be solved and failure rates brought down to reasonable levels, by moving to composite, or multi-scale indices that draw on higher resolution, localized data. The gap insurance approach pioneered in Ethiopia gives farmers recourse to a back-up village level crop cutting if they think the rainfall index fails to adequately reflect crop losses. In Mali and Burkina Faso, contract failure rates were cut dramatically through a dual-scale contract that relied on both district and village level area yield measures.
By integrating more localized measures into index insurance designs, both the Ethiopian and West African contracts require localized data, which are less likely to be available for a long time periods, and more likely to suffer missing data problems. The same problem plagues efforts to improve rainfall-based insurance contracts by installing new automated weather stations in individual localities. A denser grid of weather stations should reduce contract failure rates. Unfortunately, there is by definition a paucity of historical data for regions covered by new weather stations, making the estimation of drought and other risks less precise and more uncertain.
While especially acute for contracts based on more localized data, ‘sparse data’ (short time series and, or missing observations within a time series) is a more general problem for index insurance contracts in lower income economies. Ultimately, sparse data reflects past public good failures, which have persisted because low levels of market development, mean that there has been little demand for reliable yield and climate information. At the same time, without that information, it is hard for insurance and other markets to develop, creating a vicious circle of poor information and economic underperformance.
How then in a sparse data environment can a viable insurance market be created that offers quality contracts, at reasonable prices that are widely available? After analyzing what sparse data does to the pricing and availability of insurance contracts, this note will put forward some tentative ideas on a dynamic public-private reinsurance partnership designed to break this vicious circle.
Pricing Index Insurance in Sparse Data Environments: The Uncertainty Penalty
Figure 1 helps fix ideas by displaying a stylized index insurance contract. The horizontal axis measures the level of the insurance index that predicts farmer outcomes (yields for simplicity’s sake). When the index is at its average level (100%), yields are expected to be normal. When the index is higher, yields are expected to be above average, whereas they are expected to be below average for index levels to the left of the normal level. The figure also shows probability functions that might characterize the distribution of the insurance index.
To keep things simple, we assume an insurance contract with the following structure. As shown by the dotted indemnity line in Figure 1, when the index is above normal, no payout is given. When the index is slightly below 100% but above 75%, modest losses are expected but no payout is made as this range is the deductible part of the contract. When the index is between 35% and 75%, losses are moderate and a moderate payout of $50 per-insured hectare is made to the farmer. Finally, when the index falls below 35%, losses are severe and the farmer receives a total payout of $200/hectare.
The actuarially fair price of the insurance will depend on the probability that the index falls into the different ranges or risk layers. Let pg denote the probability that the index is above the deductible level of 75% (in Figure 1, pg is the area under the probability curve to the right of 75%). Similarly, pm denotes the probability that there are moderate (insured) losses, and ps is the probability of severe losses that trigger the large payout.
If these probabilities were known with certainty, then pricing of this hypothetical insurance contract would be straightforward. The problem, of course, is that these probabilities must be estimated using the available, often sparse, data.
Appropriate statistical methods will give us best, unbiased (point) estimates of the probabilities pg and ps. In Figure 1, we assume that the solid (green) probability curve is the best, unbiased estimate of the underlying probability structure. In Table 1, these unbiased estimates are assumed to be 25% and 8%.
While unbiased, the precision of these estimates depends on data sparseness, etc. Confidence interval estimates can be used to reflect the precision or certainty that we have about the unbiased point estimates. Continuing the example in Table 1, the interval estimate for pm might be such that we are 95% confident that the interval from 22% to 28% contains the true, but unknown value of pm. With more and better data, this 95% confidence interval estimate will shrink in size. As the data becomes sparser, the interval estimate will grow wider. The dashed (blue) curve in Figure 1 illustrates what the probability function might look like based on parameters drawn from the pessimistic end of the confidence interval for the relevant parameter estimates.
To make discussion easier, columns (B) and (C) of Table 1 show the extreme values of the interval estimates for this hypothetical example. As shown, the unbiased estimate of a severe loss (ps) is 8% (a once in very 12 year event), but that there is some chance that ps may be as low as 3%, or as high as 13%. For moderate losses, the unbiased point estimate is 25% (a once in every 4 year event), but there is some chance that it could be as low as 22%, or as high as 28%.
If we knew for sure which possible probability structure correctly described risk, we could then easily calculate the actuarially fair insurance premium (AFP) without uncertainty. The AFP is simply the product of the payout times the probability the payout occurs, summed across all possible risk layers and payout amounts. In the Table 1 example, if we knew for sure that the ‘best’ statistical estimates were correct, then the AFP would be $28.50.3 If we knew for sure that the pessimistic probability structure was correct, then the AFP would be $37.00 per-hectare insured, whereas it would be only $20/hectare under the optimistic structure. Table 1 shows the prices of the different risk layers under the different probability structures.
Table 1 makes clear, there is not only risk (the probabilities of low outcomes within a single column), there is also uncertainty about which column contains the true probability structure and risk. When data are plentiful, this uncertainty problem is trivial as it would be in the case where, say, the 95% confidence interval estimate spanned the 7.5% to 8.5% interval. However, as data become sparser, this range widens. In the Table 1 example, if the true probability structure turned out to be that represented by pessimistic column (C), then any insurance company selling insurance at an AFP of $28.50/hectare would systematically lose money. On the other hand, if the true structure were that given in the most favorable column, then the insurer would systematically make extra profits by selling insurance at the $28.50 price.
So does this uncertainty element matter for the pricing and availability of insurance? In practice, for I4 pilots at least, this is largely a matter of the pricing decisions of the private reinsurance industry. In most of these pilots, local insurance companies rely on reinsurance companies to not only price products (typically relying on data collected and analyzed by I4 researchers), but also to carry 90% or more of the risk.
At first glance, it may seem that this uncertainty should not matter. The insurance pricing problem can be looked at as a compound lottery. In the first stage lottery, the probability distribution (column) is randomly determined. As long as the first stage lottery probabilities are symmetric (as they would be under this confidence interval example), the expected AFP (averaged across first stage lottery outcomes) would remain equal to $28.50. One might imagine that this uncertainty would not matter for the pricing of insurance. What we will call an uncertainty neutral agency would price the contract at an AFP of $28.50 in this example.
However, based on experience with various insurance pilot projects, the extent of this first stage uncertainty seems to matter for the pricing of insurance by the private sector. In I4 and other insurance pilots, we have seen the AFP of insurance increased by 50%, 100% and or even more because of reaction to this uncertainty. An insurance agency that reacts this way is uncertainty averse in the sense that it prices risk using worst case scenario probability distributions rather than statistically unbiased estimates of those distributions. The additional premium charged because of uncertainty aversion is the uncertainty loading.
When the data are ample, and the uncertainty spread is modest, the uncertainty loading is trivial. However, when this statistically conservative, uncertainty-averse pricing takes place in sparse data environments, the impact on pricing can be enormous as mentioned above. This problem can be especially severe for contracts that rely on local, high-resolution data in an effort to reduce contract failure rates.
In the extreme case, when data are too sparse, private sector companies may simply refuse to offer any contract for sale in some localities. In this case, the uncertainty loading is effectively infinite—there is no price at which the contract can be bought. Several I4 projects (in Burkina Faso, Ethiopia and Mail) that had contracts of this type found that the insurance industry would not price any contract in half or more of the pilot project villages because of insufficient data for those localities.
While data interpolation and other methods of pooling data from nearby localities can be used to fill in for missing data, such statistical methods imply less precise estimates of the underlying, unknown local probability structure. In the case of I4 pilots, the insurance industry explicitly rejected these statistical methods as a way to ameliorate the sparse data problem, even when based on statistical procedures accepted by agrometeorological and other scientific communities.
Critically, the apparent uncertainty aversion of private reinsurance does not change the basic statistical properties of the problem. In the above example, selling insurance at an AFP of $28.50 would on average NOT lose money. It is based on statistically unbiased estimates. This observation suggests a role for an uncertainty neutral public reinsurance agency.
Redesigning the Public-Private Reinsurance Partnership
Observing that novel index insurance contracts were often priced at levels high enough to discourage demand and market formation, the International Finance Corporation created the Global Index Insurance Facility (GIIF) in an effort to bring down the cost of reinsurance and spur the development of viable insurance markets. Here we consider the prospects for an alternative approach to the public-private reinsurance partnership that would arbitrage between the uncertainty aversion of the private sector and the uncertainty neutrality of a proposed public reinsurance agency.
To illustrate how the GIIF currently works, and how an alternative reinsurance partnership might work, we build on the hypothetical numerical example that we have used in this brief. As illustrated in column (A) of Table 2, we assume that when the index insurance contract is reinsured by the private sector, it applies an uncertainty loading such that the AFP of the contract is $37 (Table 1) and that the market price of the contract, after a 50% mark-up, is $56.50.
Under this scenario, the GIIF could offer a subsidy to bring the contract price back down towards a more reasonable level. In I4 projects, the IFC has offered subsidies designed to bring the price down to approximately 120% of the AFP (based on the pessimistic, uncertainty averse estimates), which would be $44.40 in this example. As shown in Column (A) of Table 2, with a standard GIIF subsidy, the farmer would pay the $44.40 per-hectare insured and a subsidy of $12.10 would be transferred to the insurance industry.
Note that even with the GIIF subsidy, the farmer is paying 156% of AFP under the unbiased probability estimates. In effect, the farmer pays part of the uncertainty penalty and the insurance industry is in part being publically rebated for the uncertainty penalty. In effect, current farmers are being penalized for past public good failures that result in sparse data and uncertainty loadings.
In I4 projects, we have seen farmers balk at these kind of high prices and, or react badly to strike points (based on the worst case scenario probability estimates) that seem unrealistically low to them. Note that this approach does nothing to fix the problem of the statistical conservatism that limits market size. It also is not a cost-effective use of public dollars, as we will now explore.
Columns (B)-(D) of Table 2 consider alternative approaches to the public-private reinsurance partnership. As in the current model, a local insurance company would market and service the insurance contract. The only difference would be the nature of the reinsurance coverage.
At the heart of these alternative approaches is a public reinsurance fund that could act in an uncertainty-neutral fashion, pricing index insurance using unbiased probability estimates rather than the pessimistic estimates that seem to typify private reinsurance pricing. By arbitraging between the different uncertainty attitudes of private versus public reinsurance, these alternative approaches can realize significant cost savings. We call this uncertainty arbitrage. Rather than the uncertainty premium (and the cost of past public good failures) to the farmer, the public sector carries the uncertainty, which has zero expected cost.
Column (B) gives a first, pure uncertainty arbitrage, model of an alternative public-private partnership. Under this model, the uncertainty neutral public reinsurance firm carries the risk (and issues payments) whenever the insurance index falls into the severe risk layer. The expected actuarially fair price for this severe risk layer is $16 as shown in Table 1 when it is priced using the statistically unbiased probability structure.
Under this alternative model, the private reinsurance would price and cover payments for events in the moderate risk layer. Assuming that the private sector priced with the worst-case scenario probabilities (column (B) in Table 1), the actuarially fair price for this risk layer would be $11, giving a total actuarially fair price of $27. Assuming again a 50% mark-up over the AFP, the total market price of this contract would be $40.50.
Note that even without any subsidy, this contract would be less expensive to the farmer than the same contract costs under the current subsidy model. Note also that unlike the current partnership model, in which the initial capital of the GIIF is drawn down over time by the payment of subsidies to the reinsurance industry, this can be underwritten. Interestingly, the GIIF initially considered using its grant funds to capitalize a public reinsurance facility for index insurance. Premium for the contract would be divided between the public and private insurance companies in relation to the risks they carry. model would permit preservation of capital and a sustainable structure.
Columns (3) and (4) provide additional models that could be employed. Column (3) assumes the same expenditure (per-insured hectare) as the current GIIF model. As can be seen, this equivalent public cost reduces the cost to the farmer to almost the actuarially fair rates under the unbiased, uncertainty neutral probability structure. Given work that shows that farmers tend to be quite price-sensitive (McIntosh et al., Karlan et al.), this budget neutral, price reduction could have a major impact on demand and the development of the insurance market.
Finally, Column (4) presents a more ambitious role for the public subsidy. In this model, the public sector continues to arbitrage its uncertainty neutrality to bring down the overall cost of the insurance. In addition, in Model (D) the public sector provides all farmers, as a form of social protection, a contract covering severe events (the equivalent of the idea presented in Carter et al, 2012). In addition, farmers would be free to top up the publically provided insurance layer by buying insurance to cover moderate losses.
For the farmer who opted in and bought this second layer of insurance, the private cost would be $16. The farmer not buying the second layer of insurance would also receive the catastrophic insurance at zero cost. The public sector would be charged $24 per-hectare insured. Importantly, having public provisioning of this layer would literally build a market and also presumably generate confidence and trust in the insurance. Because it takes advantage of uncertainty arbitrage through public reinsurance of the severe risk layer, the cost of this market building, social protection strategy is substantially reduced.
Dynamics of the Public-Private Partnership
The alternative public-private reinsurance partnerships models put forward here could be designed to evolve flexibly over time as the information environment and data sparseness change. For example, in environments of extreme data scarcity, it may be that all risk layers are reinsured by the public sector. Over time, as information builds up, the intermediate, moderate risk layer could be passed to the private reinsurance sector, with public reinsurance only covering the severe tail risk.
Eventually, as additional years of data accumulate, and the interval estimates around the relevant probabilities become more tightly constructed, the entire reinsurance load could be transferred to the public sector. This model could be used in the context where new weather stations are being deployed and there is initially no data to directly price risks. Using standard meteorological algorithms, risks at the new station could be priced without bias, albeit with a level of certainty less than that which private reinsurance is comfortable.
Over time, as the new weather stations establish their own track records, risk layers could be passed to the private reinsurance sector. Similar examples could be made for area yield contracts in, say, highland Andean region where historical yield data is sparse, satellite indices are likely to fail because of pervasive cloud cover, and weather stations are likely to have especially high contract failure probabilities.
In Summary
The ideas put forward here for alternative public-private reinsurance partnerships reflect the experience of the I4 Index Insurance Innovation Initiative across a range of projects. While others may have had different outcomes than have the I4 projects, it is clear that the current system often puts the cost of past public good failure and the uncertainty loading on the low-income farmer. By dong so, this approach threatens the development of the insurance market itself.
While the public sector can offer an across-the –board subsidy designed to bring down the cost of insurance, a more cost-effective approach would be to have the uncertainty-neutral public sector reinsure those risk layers directly with a revolving fund. This would have the further benefit of allowing a greater expansion of market size (by permitting contracts with great levels of uncertainty to be sold). This would be an especially important for new generation contracts that employ designs and other technologies to downscale insurance indices and radically reduce the probability of contract failure.
In short, while the ideas put forward here would benefit from further development of public reinsurance mechanisms, it is clear that we need to move towards a model of index insurance that sustainably offers high quality contracts at prices and availability that will allow the market to develop. Without these features, it is unlikely that the promise of agricultural index insurance to resolve long-standing issues of rural poverty and low growth will remain. The goal of this brief is to stimulate a robust discussion on best to achieve this goal.
Further Reading
Carter, Michael R., Stephen Boucher and Elizabeth Long (2011). “Public-Private Partnership for Agricultural Risk Management through Risk Layering,” I4 Index Insurance Innovation Initiative Brief 2011-01
Elabed, G., M. Bellemare, M.R. Carter and C. Guirkinger (forthcoming). “Managing Basis Risk with Multi-scale Index Insurance Contracts,” Agricultural Economics.
I4 Index Insurance Innovation Initiative 2013. Insuring Against the Weather: Addressing the Challenges of Basis Risk in Index Insurance using Gap Insurance in Ethiopia, I4 Update, available at http://www.basis.ucdavis.edu/i4/publications/files/I 4%20Update%20- %20Gap%20Insurance%20in%20Ethiopia.pdf
Janzen, S. and M.R. Carter (2012). “The Impact of Microinsurance on Asset Accumulation and Human Capital Investments: Evidence from a Drought in Kenya,”
Karlan, D., Osei, R.D., Osei-Akoto, I., Udry, C., 2012. Agricultural decisions after relaxing credit and risk constraints. NBER Working Paper. Accessed February 2013, available at http://www.nber.org/papers/w18463.pdf?new_wind ow=1 .
McIntosh, C., Sarris, A., Papadopoulos, F., 2013. Productivity, credit, risk, and the demand for weather index insurance in smallholder agriculture in Ethiopia, Agricultural Economics.
Publication made possible by support in part from the US Agency for International Development Cooperative Agreement No. EDH-A-00-06-00003-00 through the Assets and Market Access CRSP. All views, interpretations, recommendations, and conclusions expressed in this paper are those of the authors and not necessarily those of the supporting or cooperating organizations. Comments encouraged: Department of Agricultural and Resource Economics, University of California Davis, Davis, CA 95616 USA ifour@ucdavis.edu http://i4.ucdavis.edu