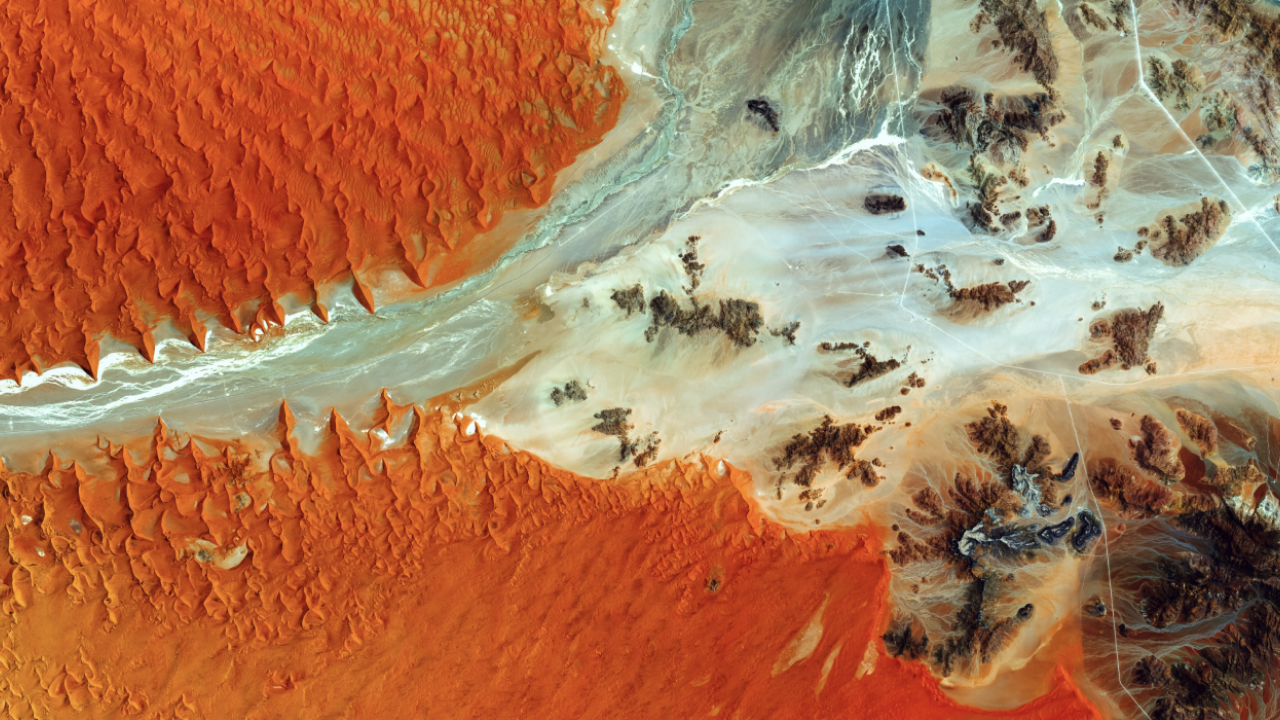
Leveraging Machine Learning to Improve Livestock Insurance for Pastoralist Families
A century ago, the thought of seeing the Earth from space might have seemed impossible. Since the 1970s, satellite technology has allowed us to measure healthy vegetation from space using bands of reflected light. This process, Normalized Difference Vegetation Index or NDVI, has been commonly used to build index insurance, a type of insurance that avoids the high costs of verifying losses individually. Since 2010, index insurance has been transformative in Kenya and Ethiopia for families who rely on livestock for their livelihoods.
Now, a new technology may be on the brink of beating NDVI on predicting forage quality, and it comes from an entirely different field. Andrew Hobbs, a researcher at the Feed the Future Innovation Lab for Markets, Risk and Resilience at UC Davis, has launched Drought Watch, a project in collaboration with Weights & Biases, a San Francisco-based startup that uses machine learning with the goal to eventually create a more accurate index than even NDVI. A more accurate measure could be used to improve the lives of pastoralist families around the world.
“We use satellite images for our index insurance already and deep learning is a type of machine learning that is really good at understanding images,” said Hobbs.
Machine Learning for Drought Protection
Pastoralist families are highly vulnerable to drought and other weather-related risks. Their livelihood depends on the health of their animals. The lack of rain where they live makes it impossible to grow crops, so they raise animals instead and move around to find quality forage. A tool to manage risk like index insurance can help these families to survive devastating droughts, as long as those products pay accurately for losses.
Hobbs is using machine learning to recognize forage quality from 100,000 satellite photographs--not NDVI measurements--of Northern Kenya collected by the International Livestock Research Institute (ILRI). NDVI averages infra-red and near infrared light into a single number. The photographs for this machine learning project are actual images.
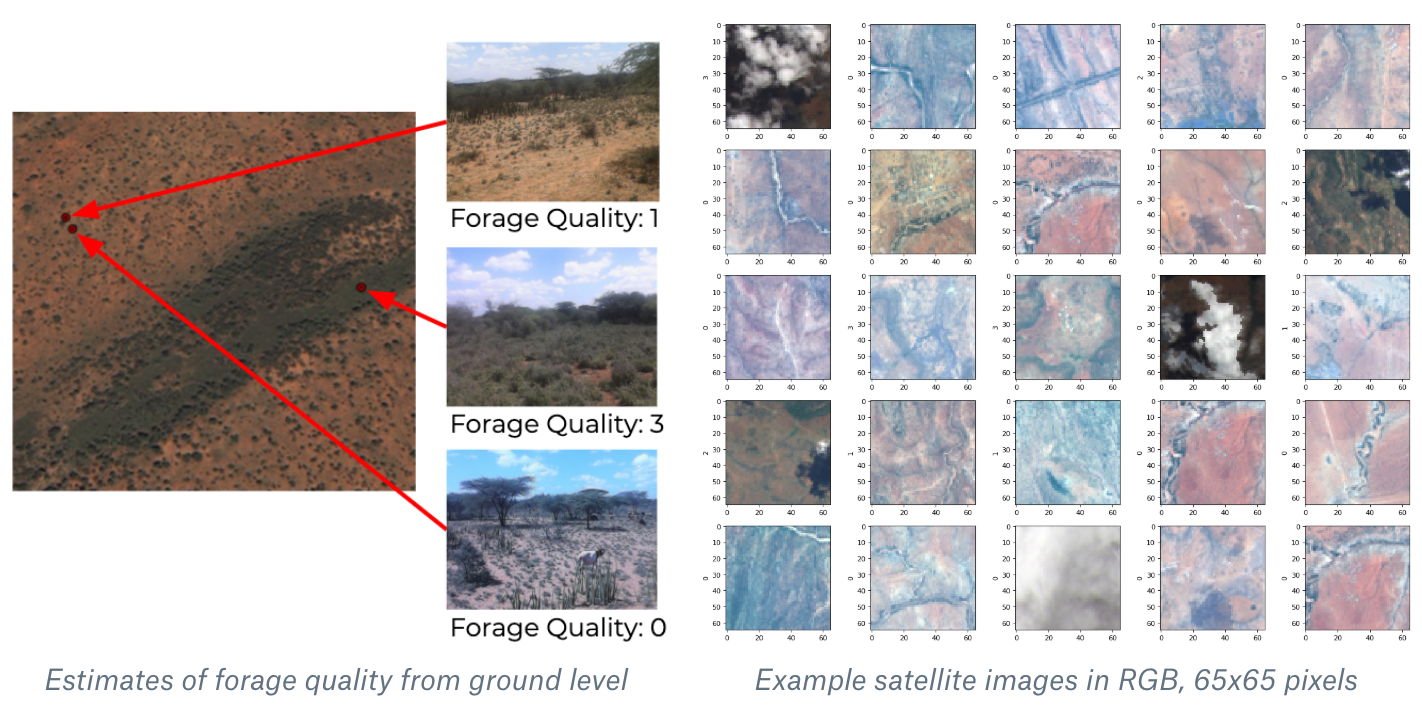
The machine learning program matches these images to data collected by ILRI from local experts who rated forage quality on the ground where the photographs were taken. ILRI developed and implemented the index-based livestock insurance in northern Kenya that was adopted by the government for the national Kenya Livestock Insurance Program (KLIP) in 2015.
Using these two sets of data, the goal is for the machine learning algorithm to recognize the forage quality in each image and then accurately predict forage quality.
“Our algorithm has more information than NDVI,” said Hobbs. “NDVI uses only the near infrared and red bands. Our algorithm can use all 11 bands of light from the image.
The machine learning technology at the center of this project has already shown extremely high accuracy with tasks such as translating French text to English and recognizing images of cats, according to Stacey Svetlichnaya, a deep learning engineer at Weights & Biases and a partner on Hobbs’s partner in the project.
But the technology is not just for picking out cats. In the field of development, machine learning has also been used for mapping poverty and studying the effects of air pollution. This project is the first time it will be used for index insurance.
For Weights and Biases, it is also the first time they are hosting a project that can be used for rural development. As a collaborative model, the project allows anyone to join and add their own code in a friendly competition to make the most accurate algorithm possible.
“I’m excited to get people to work on it,” said Svetlichnaya.
Why Index Insurance?
While Hobbs’ use of machine learning for index insurance is new, index insurance has been a tool for development for more than a decade. Michael Carter, director of the MRR Innovation Lab, has been working to improve index insurance and to study its impacts since as early as 2012.
It was then that 13,000 feet above sea level in Ecuador, Carter and his team, along with an insurance adjuster, visited multiple smallholder farms as part of a study to evaluate conventional agricultural insurance that pays for verified losses. Awake since four in the morning, they had set out to verify individual farmer’s claims.
By the end of one day, they were only able to see three farms. During their time in Ecuador, the team found that verifying one claim cost about five times as much as a single year’s worth of premiums, making conventional insurance unsustainable for smallholder farmers.
“This sounded like a great way to lose money,” said Carter
The biggest challenge with index insurance today, said Carter, is that it can be inaccurate, failing to pay a farmer who has losses or paying one who has not. A failure to pay for losses leads to deeper problems. A family who loses everything due to a weather event will also have lost the money they paid for the insurance. Additionally, insurance that takes too long to pay out will leave a family struggling.
Insurance that gives payouts quickly and accurately could make the difference between a family who overcomes a drought and a family who does not. Carter said this is why a contract that does not fail is like a “holy grail.”
The result could enhance the quality of other MRR Innovation Lab projects that rely on remote sensing, including work with The BOMA Project in Samburu county, Kenya that pairs its Rural Entrepreneur Access Project with Index-based Livestock Insurance (IBLI) from the International Livestock Research Institute (ILRI).
Potential for More Accurate Insurance
When Hobbs was first testing the potential of deep learning for index insurance, he used NDVI data to predict ILRI reports of forage quality and achieved about 65 percent accuracy. In his first attempt using machine learning with satellite photographs, he achieved 68 percent accuracy. Hobbs is hopeful that eventually machine learning will make it possible to build an index insurance that does not ever fail pastoralists when they need it the most.
It's not just the accuracy of index insurance Hobbs hopes this project will improve, but also the efficiency with which it works on the field. The algorithm can run instantly, meaning that payouts may be able to occur faster so that families are not left with nothing for long.
“As soon as the satellite identifies the drought, you could have money to them in a few hours as opposed to months,” said Hobbs.
Hobbs also said that this opportunity to use machine learning for index insurance was only able to come about at this time because of the advances technology has made in the last few years.
“It’s partly that the technology has come around and partly that we had access to the right data,” said Hobbs.
*The data used in this research was collected through a research collaboration between the International Livestock Research Institute, Cornell University and UC San Diego, and was supported by the Atkinson Centre for a Sustainable Future’s Academic Venture Fund, Australian Aid through the AusAID Development Research Awards Scheme Agreement No. 66138, the National Science Foundation (0832782, 1059284, 1522054), and ARO grant W911-NF-14-1-0498.