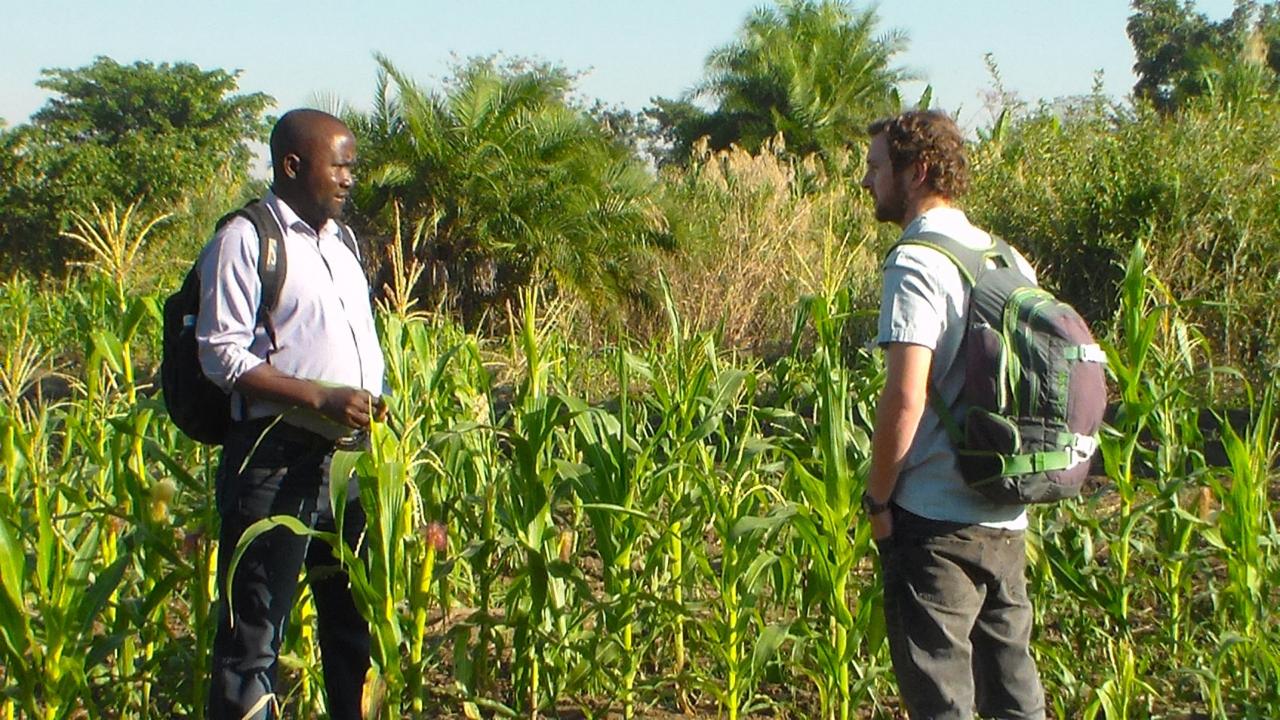
Farmers need capital, and yet lending in the agriculture sector of low-income countries is notoriously difficult. Typically, lenders have limited or even incorrect information about the creditworthiness of their customers. Often those who default on loans simply apply for new loans under different identities. When lenders respond by limiting the supply of credit, many creditworthy smallholder farmers cannot finance crucial inputs such as fertilizer and improved seeds.
Yet what if there were an affordable technology that a lender could use to improve its ability to withhold future loans from past defaulters. Presumably this lender could then find it profitable to extend more credit to more farmers. Would the lender’s ability to correctly identify borrowers and keep consistent records of their credit worthiness also have a positive impact on borrower behavior? Might they repay loans more promptly and default less? Might they be more mindful of the loan amount? Might they use the cash for inputs on the crop that effectively acts as collateral instead of diverting the loan to other uses?
This brief reports findings of a randomized field experiment that estimated the impact of biometric identification on the behavior of smallholder farmers in Malawi. The experiment used fingerprint identification, which enhances the ability of the lender to deny credit based on prior poor repayment performance and to reward responsible past borrowers with the offer of increased credit. In essence, fingerprinting allows the lender to use “dynamic incentives.” We hypothesized that fingerprint identification of borrowers can increase repayment rates, and that when farmers understand that their credit history is used as an eligibility criteria for future loans, they might become better borrowers.
Who’s Asking for a Loan?
To our knowledge, this is the first randomized field experiment examining the impact of a technology that improves the effectiveness of dynamic incentives in a credit market. We used the lender’s administrative data and a detailed follow-up survey of borrowers to estimate the impacts of fingerprinting on borrower behavior.
We collaborated with Cheetah Paprika Limited (CP) and the Malawi Rural Finance Corporation (MRFC). CP is a privately owned agri-business company that offers extension services and a package of seeds, pesticides and fungicides at subsidized rates in exchange for the commitment to sell the paprika crop to CP at harvest time. MRFC is a government-owned microfinance institution that provides financing for the in-kind loan package for up to one acre of paprika. The loan did not include cash to purchase inputs.
Instead, borrowers took authorization from MRFC to a pre-approved supplier, which provided the inputs to the farmer and billed MRFC. The loan amount was roughly 17,000 Malawi Kwacha (approximately US$120). Sixty percent of the loan went towards fertilizer; the rest went toward the input package. While all who took the loan were given the package, farmers had the option to borrow only one of the two available bags of fertilizer. Expected yield for farmers using both bags of fertilizer on one acre of land was 400-600kg, compared to 200kg with no inputs.
In the experiment, smallholder farmers organized in clubs of 15-20 members, applied for agricultural input loans to grow paprika, and were randomly allocated to either a control or treatment group. Our study sample covered four districts of the country and consisted of 249 clubs with approximately 3,500 farmers. In keeping with standard MRFC practices, farmers were expected to raise a 15% deposit and were charged interest of 33% per year (or 30% for repeat borrowers).
After we administered a baseline survey to individual farmers, we held a training session for both the treatment and control clubs on the importance of a good credit history. Then, in treatment clubs only, fingerprints were collected as part of the loan application. Our demonstration (see box) illustrated how fingerprints uniquely identify farmers for credit reporting, and emphasized that future credit providers would be able to access the applicant’s credit history simply by checking his or her fingerprint.
At this point, the names and locations of the members that applied for loans, along with their treatment status, were handed over to MRFC loan officers. Of the customers approved for loans, some failed to raise the required down payment and others opted not to borrow for other reasons. The final sample consists of 1,147 loan customers from 85 clubs. These loan customers received loan packages with an average value of US$117.
Impacts of Fingerprinting
Fingerprinting customers makes the threat of future credit denial for defaulters more credible by providing an easy, accurate way for lenders to verify the identity and credit history of loan applicants. In the absence of fingerprinting, identification of farmers relies on the personal knowledge of loan officers. This institutional memory is lost if an officer is transferred. Even when officers remain in a given location, the large number of borrowers can lead to mistakes in identification, especially in a country like Malawi where there is no widespread system of official government IDs. MRFC’s 120 credit officers handle upwards of 50,000 loans per year.
“I know your name even if you don’t tell me.”
After fingerprints were collected, we demonstrated on a laptop how we could identify an individual using his or her fingerprint. We then told the farmers: “Having a record of paying back your loans can help you get bigger loans or better interest rates. But the bank needs to know who you are so it can check if you have been a good customer in the past. Through your fingerprint, the bank will know your name, what loans you have taken, and whether you have paid them back. Having your fingerprint on file can make it easier to earn the rewards for good credit history. It also makes the punishment for not paying back your loan much more certain.”
Although loan officers were told which clubs had been fingerprinted, they do not appear to have used this information in their loan approval decisions, nor have taken treatment status into account in their interactions with the clubs. This indicates that any impacts of the treatment should be interpreted as emerging solely from borrowers’ responses to being fingerprinted.
There is no evidence that the rate of loan approval or take-up differs substantially across the treatment and control groups, on average. There is also no indication of selectivity in the resulting borrowing pool across subgroups of borrowers with different levels of predicted repayment. Yet it does appear that fingerprinted borrowers took out smaller loans—on average taking loans that were US$4.81 less than loans in the control group. Moreover, it appears that this effect was largely confined to borrowers in the lowest quintile of expected repayment, with fingerprinted borrowers in this group taking out loans roughly US$19—about the cost of half of a bag of fertilizer— smaller than those taken out by the corresponding quintile in the control group.
The existing literature tends to emphasize that improved enforcement should lead low-quality borrowers to be excluded from borrowing. Our result shows that low-quality borrowers voluntarily take out smaller loans, which leads the overall pool of money out in loans to be less weighted toward low-quality borrowers. Improvements in repayment among fingerprinted borrowers, particularly among those in the lowest quintile, may in part result from these decisions to take out smaller loans at the very outset of the lending process.
The ultimate effect of fingerprinting on loan repayment was highly encouraging. Results show that fingerprinted borrowers have lower outstanding balances, have higher fractions paid, and are more likely to fully pay on time as well as eventually. The effect is largest for those borrowers with the lowest predicted repayment rates absent any intervention. The magnitudes of the repayment effect for this subgroup are large. The approximately US$50 effect on eventual outstanding balance amounts to 40% of the average loan size for these borrowers.
Better Farming Practices
We examined farmer decisions throughout the planting and harvest season that may contribute to higher repayment among fingerprinted farmers. One of the first decisions that farmers make in any planting season is the proportion of land allocated to different crops. Land allocation decisions may be affected by fingerprinting because the lender can only feasibly seize paprika output and not other types of crop output.
By not producing paprika (or producing less), the borrower is better able to avoid repayment. By increasing the incentive to repay loans, fingerprinting seems to discourage diversion of land to other crops. Again, for the group in the lowest quintile of predicted repayment, fingerprinting has the largest impact. Our data indicate that fingerprinting leads farmers to allocate 8.3 percentage points more land to paprika. This effect is only marginally statistically significant, but it is large: roughly half the size of the paprika land allocation.
The other major farming decision is input application, which takes place later in the agricultural cycle than land allocation. Agricultural inputs are more fungible than land, and inputs are added multiple times throughout the season, so farmers can incorporate new information about the cost of default into their use of inputs but cannot change land allocation after planting. Thus, use of inputs should respond more quickly to the introduction of fingerprinting than would allocation of land.
Indeed, particularly for those same farmers expected to have a lower likelihood of repayment absent the treatment, fingerprinting leads to higher application of inputs and less likelihood that these farmers dispose of the inputs via sale or barter. In this low-quality subgroup, fingerprinted farmers used roughly US$45 more paid inputs in total, which is substantial compared to the mean in the lowest predicted-repayment subgroup of approximately US$51. The most likely scenario is that, in the absence of fingerprinting, borrowers in this riskier subgroup were not using the inputs received as part of the loan for paprika production. Rather, they most likely sold or bartered them away. When such borrowers were fingerprinted, they became more likely to use the inputs as intended, expanding land allocated to paprika and using the inputs on that crop as the loan required.
Given these effects of fingerprinting on land allocation and input use, it is feasible that fingerprinted farmers will ultimately enjoy higher revenues and profits. However, our data do not allow us to make strong statements about the impact of fingerprinting on farm profits.
Cost Benefit
We have seen that a financial institution can profit from using fingerprinting, but these gains need to be weighed against the costs of using this technology. We consider three general types of costs: equipment, loan officer time, and the transactions costs of checking fingerprints. Taking into account these costs, the net benefit per individual fingerprinted is US$1.84. The benefit-cost ratio is an attractive 2.27.
This benefit-cost calculation is likely to be quite conservative. The cost for equipment units could fall substantially if a fingerprinting function were integrated into equipment packages that had multiple functionalities, such as the hand-held computers that MRFC is considering for all of its loan officers. Transactions costs for fingerprint checking could fall due to volume discounts if the lending institution joined other lenders to channel all fingerprint identification through a single service provider.
There are other benefits to the lending institution that our benefit-cost calculation did not capture. The impact of fingerprinting on loan repayment may become larger over time as the lender’s threat of enforcement becomes more credible. The benefits our study found were from fingerprinting new loan customers, but there also may be increases in repayment among existing customers who undergo fingerprinting. Finally, there may be welfare benefits that go beyond the profit of the lending institution, such as increased income for farmers due to more intensive input application.
Broader Welfare
Fingerprinting led to substantially higher repayment rates for the subgroup of farmers who would have been considered the highest default risk prior to the experiment. Not only do these farmers take out smaller loans, they also devote more land to paprika and apply more inputs on the paprika crop. In this way, loan repayment improves because farm output improves, giving farmers higher incomes with which to make repayments. By contrast, we found that fingerprinting had no impact on repayment for the rest of the farmers.
There are reasons to expect the short-term improvements in repayment would expand over time. Why? Borrowers’ assessments of the effectiveness of the technology and the credibility of the threat to withhold credit would likely rise over time as they gain further exposure to the system, observe that their past credit performance is being correctly retrieved by the lender, and see that their credit history is indeed being shared with other lenders.
In addition, the lender should be able to selectively allocate credit to the pool of good-performing borrowers, further improving overall repayment performance of the borrowing pool. Finally, because there is less risk involved for the lender, the credit contract terms could be made more attractive to borrowers, which may further improve repayment rates.
Beyond improving the profitability and financial sustainability of microlenders, increased adoption of fingerprinting (or other identification technologies) can bring additional benefits if lenders are encouraged to expand the supply of credit, and if this expansion of credit supply has positive effects on household wellbeing. Credit expansions enabled by improved identification technology may be particularly large in previously underserved areas, such as other rural sub-Saharan African countries, where problems with personal identification are particularly severe.
Another potential implication of this research is that, in the absence of an alternative national identification system, fingerprints could serve as the unique identifier that allows individual credit histories to be stored and accessed in a cross-lender credit bureau. It has been noted that a key obstacle to the establishment of credit bureaus is the lack of a unique identification system. Our results indicate that borrowers (particularly the worst borrowers) do perceive fingerprinting as an improvement in the lender’s dynamic enforcement technology.
The effects of fingerprinting on repayment could very well rise over time, and so future studies should monitor effects beyond a single loan cycle. Future work should also examine responses by the lender, such as changes in the credit contract, approval rates or in loan officer monitoring.
Related Reading
Chiappori, P-A., B. Jullien, B. Salanié and F. Salanié. 2006. “Asymmetric Information in Insurance: General Testable Implications” Rand Journal of Economics 37: 783-98.
De Janvry, A., C. McIntosh and E. Sadoulet. Forthcoming. “The Supply and Demand Side Impacts of Credit Market Information.” Journal of Development Economics.
Giné, X., P. Jakiela, D. Karlan and J. Morduch. Forthcoming. “Microfinance Games.” American Economic Journal: Applied Economics.
Giné, X. and D. Yang. 2009. “Insurance, Credit, and Technology Adoption: Field Experimental Evidence from Malawi.” Journal of Development Economics 89: 1-11.
Karlan, D. and J. Zinman. 2009. “Observing Unobservables: Identifying Information Asymmetries with a Consumer Credit Field Experiment.” Econometrica 77(6): 1993-2008.
Ligon, E., J.P. Thomas, and T. Worrall. 2002. “Informal Insurance Arrangements with Limited Commitment: Theory and Evidence from Village Economies.” Review of Economic Studies 69(1): 209-44.
Publication made possible by support in part from the US Agency for International Development Cooperative Agreement No. AEG-A-00-08-00008 through the Assets and Market Access CRSP. All views, interpretations, recommendations, and conclusions expressed in this paper are those of the authors and not necessarily those of the supporting or cooperating organizations. We especially thank Santhosh Srinivasan, Lutamyo Mwamlima, Ehren Foss, and Niall Keleher for their work.