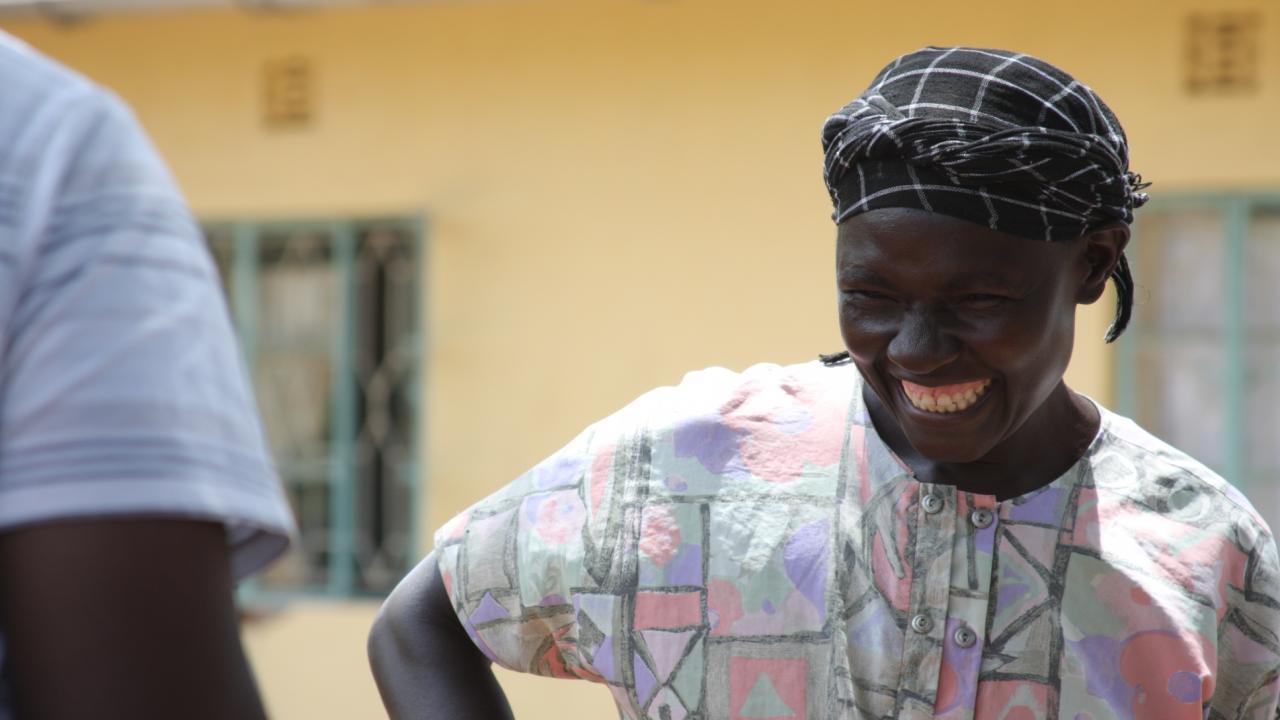
Approximately three-quarters of poor people in developing countries live in rural areas and depend at least in part on agriculture for their livelihoods. Yet, despite many advances in agricultural technology, smallholder farmers still suffer from low productivity, which often leads to chronic poverty and food insecurity. These improved technologies could raise agricultural productivity to both lift these poor households out of poverty and grow the economies in which they live, but adoption has been slow in poor countries, especially sub-Saharan Africa.
Key Points
- Social network effects are weaker in villages that are more heterogeneous.
- The more variable the environment, the more important learning-by-doing becomes. Seed packet recipients were 10% more likely to purchase and plant the seeds in the next main season.
- In areas where soil type varies significantly across farms, policy-makers should consider focusing attention (and subsidies) on encouraging learning-by-doing, while in homogenous areas they might get bigger impact on the by leveraging social learning.
Understanding how farmers make their production choices is essential to designing effective interventions to promote new agricultural technologies to close yield gaps and reduce poverty. In particular, why don’t smallholder farmers adopt technologies that have the potential to boost farm productivity and improve their household’s welfare?
One reason is that the market in which farmers make their choices is plagued by imperfections. The challenges faced by farmers include credit constraints, imperfect insurance markets, and incomplete information about the availability and profitability of new technologies. This research focuses on the last of these challenges, and examines under which circumstances social learning can play a role in diffusing information about a new agricultural technology.
The Hybrid Seed Market in Kenya
A partnership with a private seed company in Kenya provides a promising opportunity for BASIS researchers to attempt to answer the question, “what role does social learning play in the adoption of improved agricultural technologies?” The data for this study comes from a subsample of the study population of a large-scale randomized control trial (RCT) that aims to evaluate the socio-economic impacts of a new hybrid maize seed that is produced by Western Seed Company (WSC). The main study is built around a randomized roll-out of information about and samples of the company’s high-yielding maize hybrids. Until recently, the company faced production capacity constraints and therefore had a limited geographic reach. As a result, prior to this study, farmers in the study areas had neither been exposed to information about these new hybrid seeds nor had a chance to use them.
Unlike many other parts of sub-Saharan Africa, hybrid seed use among maize farmers in Kenya is quite high. Because of this, it may seem like an unusual setting in which to study learning about maize hybrids as a new technology. However, some blame Kenya’s stagnating maize production on how slowly older hybrids are being replaced with newer releases. The relevant decision for farmers is therefore not simply whether to plant a hybrid, but what type of hybrid to choose. In contemporary Kenya, an average of over fourteen new maize varieties have been released on the market each year since 2000, making this a very complex choice. The choice is made even more difficult by the fact that the region being studied is characterized by significant differences in soil quality both within and between villages.
A New Way to Measure Social Network Effects
The study of social learning has grown in popularity over the past few decades, but can be difficult to properly identify. Similar behavior among members of a social network, for example, does not necessarily mean there is social learning in the group. The trick is to identify whether members of a social network influence each other or whether they behave alike simply because they are alike and in similar situations and environments. Most modern papers experimentally vary the information available through social networks to cleanly pick up the effects of social networks. They then base their main social network analysis on the number of members of an individual’s network that were treated/received a piece of information (as a proxy for the number of different sources of information to which a farmer has access).
BASIS researchers complement these prior methods with more precise measures of the information available through farmers’ social networks. As part of the experiment, villages were randomly designated as either control or treatment villages. Importantly, in treatment villages only those farmers randomly selected for inclusion in the study were actually treated. Before the main planting season of 2013, the farmers selected for treatment were invited to an information session and given a 250-gram sample pack of the new seeds. In early 2014 researchers conducted a phone survey with treated farmers to learn more about their experience with the sample seeds. Researchers explicitly elicit farmers’ experiences with the technology and obtained the treated farmers’ evaluation of how well the on-farm experiment went.
Researchers used this information on their experiences to construct a measure of the information flow through the network, specifically the percentage increase of WSC hybrid harvest over the expected harvest with seeds the farmer would have normally used. They then let the signal that a given farmer receives about the new technology be a function of the distribution of these evaluations in her information network.
The information networks were obtained through an add-on network survey in a sub-sample of twenty treatment villages in Western Kenya. All of the treated farmers in a village were invited to an experimental session together with a random sample of their fellow (untreated) villagers. These untreated fellow villagers residing within treatment villages are referred to as “indirectly treated.”
After eliciting some basic information about the household and farm, the farmers were presented with a network module, in which they answered a variety of questions about the approximately 30 farmers in the session. The questions asked them to identify relatives, who they speak to about agricultural issues, geographic proximity, and perceived similarities. Another set of questions measured their knowledge about other farmers in the experimental sample (such as what maize seeds they planted and whether they would recommend WSC hybrids).
Observing peer effects may reflect mimicry or social pressure rather than actual learning, but these more precise measures of information enable us to more carefully discern between imitation and actual learning. In this study, researchers contrast individuals’ response to the number of people who have experience with the new technology with their response to the actual information being transmitted through the network (the signal described above). If people respond to the number of people in their network who adopt a new technology, but not to information about the returns to this technology, then any observed effects from the social network are likely to be a sign that mimicry, rather than social learning, is at work.
The Role of the Social Network in Learning
Researchers found that the information signals impact the indirectly treated farmers’ familiarity with the new technology, their willingness to pay for the seeds, and their probability of adopting the new technology. For treated farmers, social networks have only moderate impact on individuals’ familiarity with the technology, but they affect farmers’ willingness to pay for the new seeds as well as their probability of adoption.
Researchers also find that the observed social network effects are weaker in villages in which soil quality is more varied. Finding that this heterogeneity handicaps social learning also gives researchers additional confidence that the social network effects observed are due to learning rather than imitation. It is unlikely that researchers would observe a negative relationship between unobserved soil heterogeneity and social network effects if farmers were merely imitating their peers. Researchers conducted the bulk of their analysis of social network effects using four main outcome variables:
- Familiarity with WSC hybrids,
- Willingness to pay for WSC hybrids,
- Whether they planted a WSC variety, and
- Whether they planted a non-WSC variety.
Heterogeneity, Network Effects, and Learning
To study the impact of unobserved heterogeneity on network effects, researchers used detailed soil quality data on the treated farmers’ fields. When asked to identify the farmers in the sample who have similar soils to theirs, more than half of the sample identified only one other farmer and 14 percent of the respondents stated they did not know whose soil was similar to theirs. For those with smaller networks, the number of people treated in their network has a significant positive impact on respondent familiarity with the new technology. However, as a respondent’s network size grows, the number of treated in the network becomes less important.
While the results above show that social network effects influence behavior in the sample villages, observing or talking to one’s neighbor may be more or less useful depending on how similar they are along dimensions that matter for the profitability of the technology. It is harder for individuals to learn from their network members about a technology that is sensitive to unobserved characteristics (such as soil quality) if those characteristics vary in the population. Large variation in unobserved characteristics, like soil quality, could negatively impact social learning.
At low levels of soil quality variation, the average information signal in an individual’s network positively influences all four outcome variables. As the variation increases, the impact of the average signal decreases. Figure 1 (left) shows this effect for willingness to pay. The x-axis shows the variability in soil quality, and the y-axis displays the effect of the average information signal on individuals’ WTP. For the treated, the impact of soil quality variation is less pronounced, suggesting that having personal experience with the technology may help to mitigate some of the negative impacts of unobserved heterogeneity. These results suggest that farmers are aware of this particular type of heterogeneity and that it affects how much they can learn from their social contacts.
Policy Recommendations
BASIS researchers have identified strong evidence of information passing as well as social learning in the adoption of agricultural technologies. In villages where farmers have very different soil quality from one another, researchers found that the network effects (learning from others) are significantly weaker than in villages where soil quality is similar across farms. In other words, for a farmer in the developing world it is hard to infer about the returns to a new technology on your own soil from observing the returns on soils that are very different from your own.
A better understanding of the complexities that farmers face when making input decisions is key to understanding why some innovations diffuse more slowly than would be socially optimal. Farmers react to unobserved heterogeneity by relying less on information from their peers when making agricultural decisions. The more variable the environment, the more important learningby-doing becomes. In this study, seed packet recipients were much more likely – ten percentage points – to purchase and plant the seeds in the next main season.
This implies that in areas where soil type varies significantly across farms, policy-makers should consider focusing their attention (and subsidies) on encouraging learning-by-doing, while in homogenous areas they might get bigger impact on the same spending by leveraging social learning.
Further Reading
Tjernström, E., 2015. Signals, Similarity and Seeds: Social Learning in the Presence of Imperfect Information and Heterogeneity. Mimeo.
Carter, M.R., Laajaj, R., Yang, D., 2014. Subsidies and the Persistence of Technology Adoption: Field Experimental Evidence from Mozambique (Working Paper No. 20465). National Bureau of Economic Research.
Munshi, K., 2004. Social learning in a heterogeneous population: technology diffusion in the Indian Green Revolution. Journal of Development Economics 73, 185–213.
Publication made possible by support in part from the US Agency for International Development Cooperative Agreement No. AEG-A-00-08-00008-00 through the Assets and Market Access Collaborative Research Support Program. Funding for this project was provided by the United States Agency for International Development. All views, interpretations, recommendations, and conclusions expressed in this paper are those of the authors and not necessarily those of the supporting or cooperating organizations.