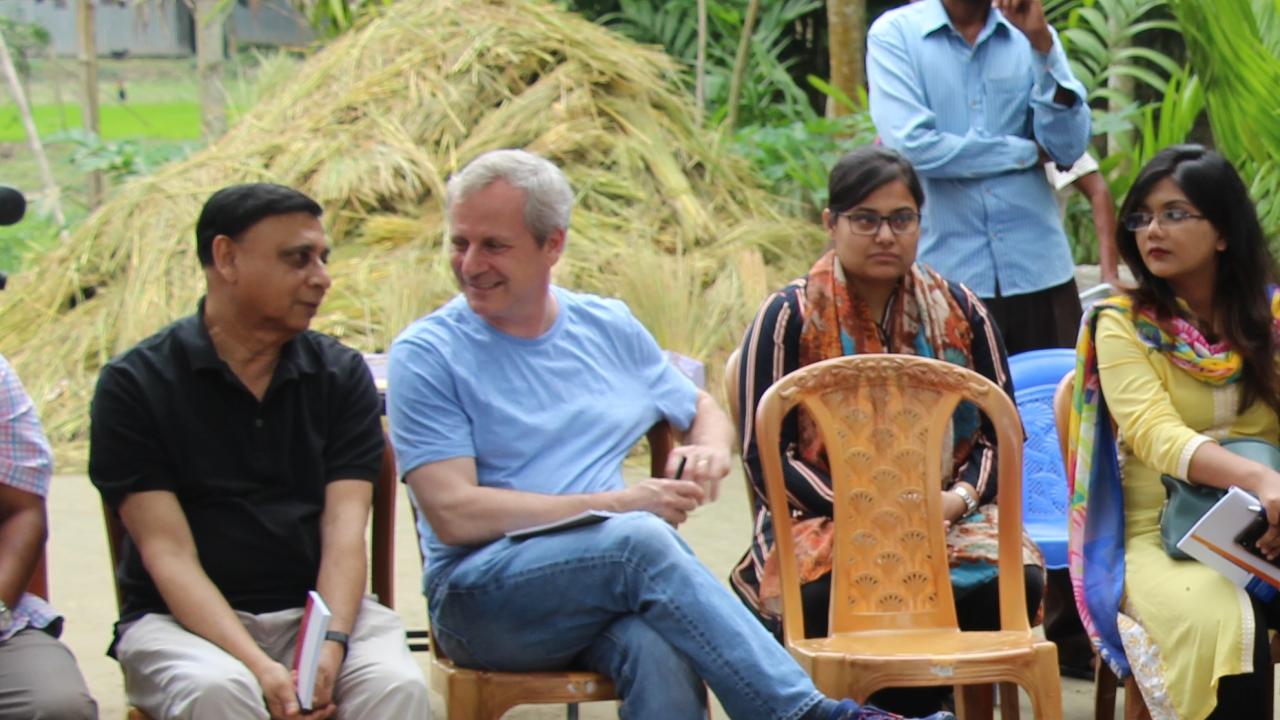
Q&A: John Hoddinott on Shock-Resilient Impact
John Hoddinott is the H.E. Babcock Professor of Food and Nutrition Economics and Policy, Cornell University. His research focuses on the causes and consequences of poverty, hunger and undernutrition in developing countries.
Together with researchers at IFPRI, John has recently completed two MRR-funded studies that study the long-term impact of interventions four or more years after their end—and following shocks, including Covid-19 lockdowns. The ANGeL and TMRI interventions both sought to improve household nutrition in rural Bangladesh, and both achieved sustained impacts among some treatment groups over time and amid shocks. ANGeL took an exclusive training approach, while TMRI offered cash and food transfers and offered training only to some treatment groups.
In this conversation, John elaborates on key takeaways from the studies, methods for collecting data amid a shock, and different ways of measuring resilience. He also discusses quandaries around allocation of limited resources—such as whether programs should use limited funds to maximize reach or focus on impact among a smaller group, and what balance of specializing vs. self-provisioning is best amid the quest for economic development in a shock-prone world.
For TMRI, you found that women in households that received cash transfers and nutrition behavior change communication (BCC) started livestock rearing, which appeared to improve their household’s food security, even though this livelihood strategy wasn’t specifically encouraged in the BCC component. What are your takeaways from this result?
We were surprised that people did this!
This program was focusing on a very poor population, but giving them meaningful amounts of resources over a relatively long period of time. This takes us to a difficult tension: We have a certain budget with which we can assist a group of people in a particular area. We could spread that widely—which might be more equitable, but it might not have sustainable effects. Or we could concentrate it among a small number of people and be somewhat more confident that the effects are going to last after we leave. That is a really hard question that I’m not sure we economists actually think hard enough about.
What is a common takeaway you see in the results of both the ANGeL and TMRI studies?
One of the things we see is that a combination of activities seems to be more powerful than a single activity. In ANGeL, there’s evidence that combining the agricultural and nutrition training interventions is more powerful than either on its own. In TMRI, providing cash plus BCC seems to be more powerful than cash on its own. And why might that be?
In both programs, there is something that people can run with after the intervention ends. It may include increased liquidity, new skills, and possibly—I’m speaking speculatively here—a slightly different way of looking at the world. This notion, “I can undertake actions, I have more agency than I realized.” Trainings in both programs had a common element of working through problems and how to solve them.
It's also worth noting that these activities occurred in physical places where people could act on these ideas. The original TMRI intervention was also done in southern Bangladesh, but we didn’t see any persistent effects from that intervention. Southern Bangladesh is not a great place for raising livestock; it’s prone to flooding, and therefore has high soil salinity as well as periodic floods that drown the livestock. So having a physical place where you can put ideas, like raising livestock, into action is an important component of this combination of elements that can create powerful, long-term effects.
Let’s talk about trying to collect data amid a shock. Your team used phone surveys as a workaround for data collection when Covid numbers were high and travel difficult. What did you observe about the phone surveys compared to the traditional, in-person method?
We learned from the Covid-19 pandemic that one can get reasonably useful information in the context of a short phone survey, particularly on well-defined welfare measures. But we also had the experience that you can only ask so many questions. After about 10 or 15 minutes, respondents’ attention drops off, and I don’t see any real way of extending that. Whereas in a face-to-face survey, your enumerators and respondents can talk for 20 minutes, then everybody can get up and walk around, and then go back and do some more questions.
With a longer, in-person survey, you can also triangulate the information. Not only can you ask more questions, but you can look at the context around you. For instance, if you see several cows, you might prompt them on income they make from selling milk.
You use several different frameworks for measuring resilience across these two papers. For ANGeL, you adapted the Livelihood Coping Strategy Index to assess households’ resilience based on what coping actions they took amid shock—for example, did they reduce essential expenditures or sell productive assets. Could you talk about how you measured resilience differently in TMRI, and what takeaways you have from using these different measures?
For TMRI, we don’t have access to data amid the shock, but we assess resilience before before and after the shock. We can look at pre-pandemic accumulation of livestock and savings as building up resilience “capacity.”
Unfortunately we don’t have data for the period when the shocks were occurring – when lockdowns were in place. But after the shocks, we look at food security as our measure of people’s resilience. We use a score of four on the eight-point Food Insecurity Experience Scale (FIES) as a normative threshold – we consider households with a score under 4 to be resilient.
We are working on a paper using different measures from TMRI data. We’re going to look at the predictive power of different variables on resilience and do a comparison across them. As you know, some measures to measure resilience are very complicated. One of our questions is, do the complicated measures lend more accurate estimates of resilience?
At this moment in time, I do not have the answer to that question. I have a deep suspicion, however, that a lot of resilience comes down to human capital and level of access to income generating resources as the main drivers. And if that’s the case, maybe we don’t need these more complicated measures.
One idea we have goes like this: In terms of household asset ownership, more and different assets suggest that you’re wealthier relative to someone who has a smaller number and variety of assets. So the number of different assets you own could be a proxy for your level of wealth. That’s one of the proxies we’re going to look at and see whether it does a good job predicting whether a household maintained food security.
Bangladesh sees a lot of natural disasters. What implications did Covid-19 lockdowns bring compared to other shocks?
A real problem with the lockdown was even if people had money, they couldn’t spend it, as markets were locked down. But if you have another way of producing food for your household, you could do that while the lockdown was taking place.
We economists are really tied to the notion of comparative advantage. People should specialize in what they’re relatively good at, and we want to create conditions where people can do that. So we don’t necessarily want people to focus on becoming self-sufficient with food production. It’s a goofy example, but I know I’m good at economists and I’m really terrible at farming. It would make no sense for me to try to self-provision on food.
But at the same time, we have this extraordinary event—the Covid-19 pandemic—and it turns out all of a sudden that self-provisioning is actually really helpful. Some TMRI treatments enabled people to be better at self-provisioning, which meant they were far less likely to be food insecure. So as we think to the future, how do we balance the productivity gains realized through exploiting comparative advantage with the importance of providing downside protection to households?